Alarming Bias in Predictive Policing
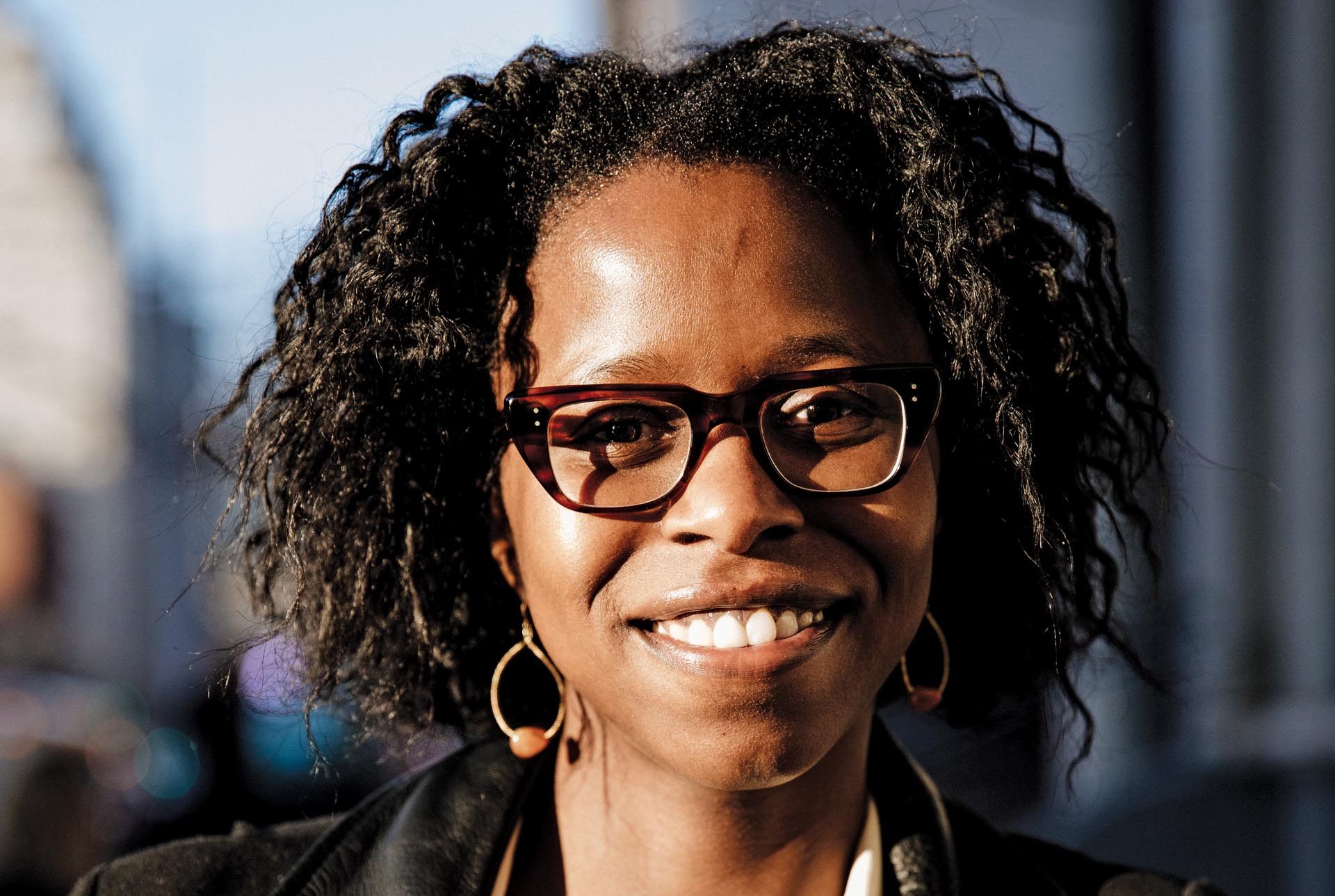
Rashida Richardson ’08, director of policy research at the AI Now Institute at New York University, examines the underlying assumptions in so-called “neutral” data sets.
Predictive policing technology uses two models: the person-based model tries to identify people who might be either the victim or perpetrator of a crime; the place-based model uses historical data to predict where and when a crime might be most likely to occur.
The problem with both these models is that they are largely trained, developed, and run on police data. While we’ve seen a lot of media coverage on the racial bias inherent in person-based systems, we’ve also heard it suggested that place-based systems are more neutral. But the data for both is derived from the same place: local police departments, as dictated by the local politics and incentives of that place and time.
In New York City, for example, place-based data points include complaint calls to 311. Those calls are often considered “objective,” as they come from a number of sources. But I would argue that even these data points are skewed. Research shows that when a neighborhood is becoming more gentrified—specifically when there’s a large influx of more affluent and white residents—there’s a huge uptick in those 311 calls.
Is the neighborhood noisier or have the expectations changed? Perhaps people aren’t comfortable talking to their new neighbors, so they are calling the police instead. A recent report also documents that after the neighborhood has experienced a large influx of white residents, 311 calls are three times more likely to result in an arrest. While that survey didn’t collect demographic data on those detained, the presumption is that the arrests disproportionally affected the older residents, who are more likely to be lower-income people of color as the targets of these calls.
This is place-based data embedded with bias.
When we’re putting technology in social domains, we have to realize that it not only affects the person who is specifically targeted in that one incident. It also begins a feedback loop into society, setting up expectations on that neighborhood based on the data that comes from this event.
Before we employ the kind of artificial intelligence used in predictive policing, the most important questions I would ask are: 1) What is the problem we’re trying to solve? and 2) Is this technology actually a solution for that problem?
If the goal of technology for predictive analytics is public safety, then the real answer might be to increase public funding for services that actually have been proven by research to help reduce public safety issues. Crime often results from the lack of government investment in social systems that people need to survive.
Those in politics or local government might find it tempting to be upsold on this technology by a company that promises, “We can help reduce your crime rates.” Instead, I’d suggest those leaders engage in conversations with the community and constituents. Ask people: “How do we use our limited budget to help fill the gaps, to provide the social support that communities may need to function better?”
Those dialogues might be the harder, but more enduring route to real improvement in society.
The above was adapted from a conversation with the editors. A College of Social Studies major while at Wesleyan, Richardson graduated with honors and earned her JD from Northeastern University School of Law. Read her New York Law Review article here: “Dirty Data, Bad Predictions: How Civil Rights Violations Impact Police Data, Predictive Policing Systems, and Justice.”