Microsoft Data Scientist Jennifer Chayes ’78 Elected to National Academy of Sciences
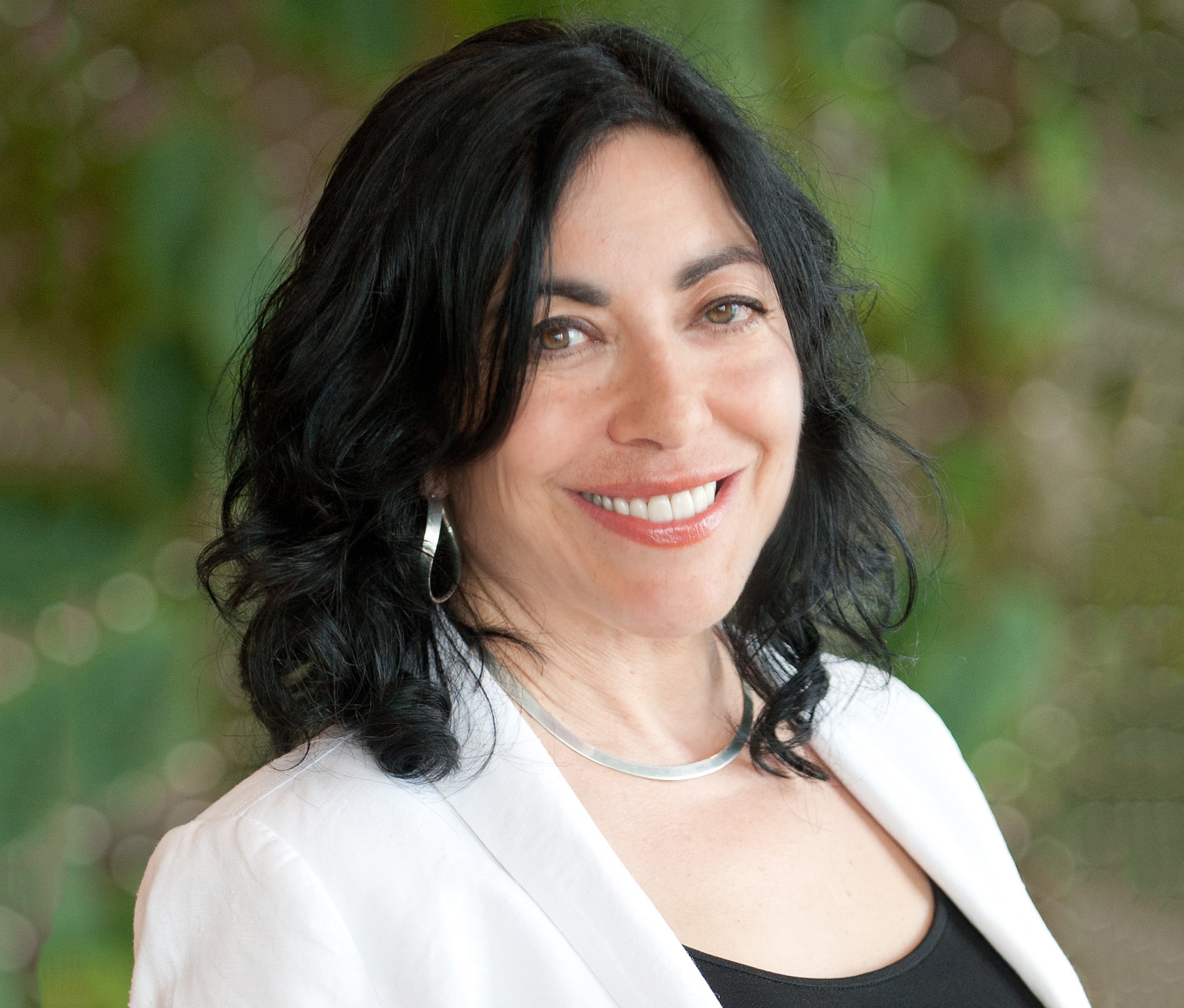
The head of Microsoft Research New England, NYC, Montreal, Jennifer Chayes brings FATE—fairness, accountability, transparency, and ethics—to data science. Her own unexpected path to the field has sensitized her to bias.
On April 30, The National Academy of Sciences announced the election of 100 new members and 25 foreign associates to its ranks, recognizing distinguished and continuing achievements in original research. Included in the roster is Jennifer Chayes ’78, technical fellow and managing director of Microsoft Research New England, New York City, and Montreal.
The academy noted that 40% of these newly elected members are women, historically the most ever elected in any one year.
Chayes, a physics major as an undergraduate at Wesleyan, holds a doctorate in mathematical physics from Princeton University and was previously professor of mathematics at the University of California, Los Angeles.
A 2016 podcast from a Women in Data Science (WIDS) conference provides an interview with Chayes by conference cochair Margot Gerritsen, Stanford University professor of energy resources engineering and senior fellow at the Precourt Institute for Energy.
With Gerritsen, Chayes discussed her career and fortuitous (but perhaps surprising) path.
Data scientists, she tells Gerritsen, have “the opportunity to build algorithms with fairness, accountability, transparency, and ethics, or FATE.” She notes that the group at her lab who formed around those concepts are diverse individuals, some with unlikely journeys to their career in data science.
“You are much more likely to see a lack of fairness, inherent bias, as a problem if that has been your experience,” she tells Gerritsen. “You are much more likely to ask the right questions if you have been on the wrong side of outcomes.”
For instance: Chayes notes that widely used artificial intelligence algorithms have “learned” gender bias from data. Using these algorithms to complete analogies can give biased results. Consider the analogy: “Man is to woman as [something] is to [another thing].” Given the word “king” the algorithm would be correct in completing the comparison with “queen.” But, given the word “doctor,” the algorithm returns the word “nurse,” an incorrect and biased solution: neither profession has a dictionary definition that includes a gender assignment. Chayes notes that a researcher in her lab found a way to remove this gender bias from the algorithm. When the gender-scrubbed algorithm was fed into search engines or job matching sites, it returned fairer results, e.g., a help-wanted ad for a computer programmer began being offered to people with both male and female names, rather than only male names.
Other times, Chayes observes, gender skews outcomes in ways that call for adjustments in applying criteria. She considers this hypothetical example: What if she were to determine a list of characteristics, qualities, and background information that correlated with success in a technology company, which has many more male employees? And what if one item that seemed linked to lack of success was a gap in employment a few years prior to hiring? But, she queries, what if a gap in employment was correlated in different ways for male and female employees? Perhaps female employees with a gap of a few years tend to be people who took time off to have a family and returned with great multitasking skills, which positively correlated with success? The data of female employees would be overwhelmed by the data of the male employees since there are many more men. We need to explore the biases inherent in the data before applying them across the board, she says.
“There is an opportunity for us as we look at our data and as we come up with our algorithms to ask ourselves: How do we make them fairer than human beings?” she says. “Just as we are trying to make our self-driven cars safer than human-driven cars, we should make our artificial intelligence both fairer and safer than our human decision makers.”
What Are the Chances?
The story of Chayes’s path to Microsoft emerges from mapping five key influences along the way.
“I don’t come from a mathematical background,” Chayes says of her family. Instead, she traces an unusual—some might say “unlikely”—path to Microsoft Research New England, New York City, and Montreal.
1. It was the neighbors, not her family, who initially introduced her to the fun of mathematical problem-solving.
As a little girl, Chayes, with her younger brothers in tow, used to ring the doorbell of a neighbor, a kind woman who would hand out treats. Peering inside the home, though, Chayes could see the husband and their 20-something daughter, talking and laughing while they did math problems. “May I have a math problem instead?” Chayes asked. Amused, the neighbors obliged and the little girl became a regular around the neighbors’ math table. “They were my inspiration,” she says.
2. A junior high math teacher showed her that geometry was visual, a fun field of study.
As a preteen Chayes was deciding whether to focus on art or mathematics. When her math teacher started introducing geometry and proofs, Chayes had her answer: “The fact that I could create . . . structures with math convinced me that I could ‘paint’ with math. It convinced me that math was creative.”
3. Chayes dropped out of high school.
Instead of the local public school, Chayes attended an alternative high school in a church basement, with adult volunteers teaching the major subjects, except for one: “We had nobody to teach math, so I’d learn it and then teach it to the other students,” she recalls. She also took some calculus classes at a local community college. “I loved math; it came easily to me,” she told Gerritsen.
4. At Wesleyan, she expected to major in biology and become a doctor.
As part of the premed curriculum, she took an introductory physics course: “I absolutely fell in love with physics and decided I wanted to do mathematical physics.”
5. With a PhD from Princeton in mathematical physics and tenure at UCLA, she gave up her position in academia to set up a lab at Microsoft.
A professor at UCLA for about a decade, Chayes was eager for interdisciplinary work but didn’t initially take Microsoft seriously when they reached out with an offer to set up her own lab, especially since “My last class coding had been in a class as a freshman, in Fortran and Pascal.” They asked what she wanted. An interdisciplinary group with mathematicians and physicists, she told them. They agreed.
Says Chayes, “There are brass rings that come along and they come along at the most inopportune times, and they look really scary, but I believe that we should grab them.”
The recipient of numerous leadership awards, including the Anita Borg Institute Women of Vision Award and the Mass Technology Leadership Council Distinguished Leader Award, Chayes focuses her recent work on machine learning, including applications in cancer immunotherapy, ethical decision-making, and, most recently, climate change. She received an honorary doctorate from Leiden University in 2016.
Read an earlier Wesleyan magazine article about Jennifer Chayes, “Lab of Ideas.”